JENNIFERCLAAR
I am JENNIFER CLAAR, a high-energy astrophysicist specializing in decoding the evolutionary pathways of supernova remnants (SNRs) through multi-wavelength morphological analysis. With a Ph.D. in Stellar Death Dynamics and Computational Astrophysics (California Institute of Technology, 2021) and a NASA Einstein Fellowship at the Harvard-Smithsonian Center for Astrophysics (2022–2024), I have revolutionized SNR taxonomy by integrating turbulence theory, magnetohydrodynamic (MHD) simulations, and machine learning. As the Principal Investigator of the Galactic Supernova Atlas Project and Lead Scientist for the ESA’s ATHENA X-ray Observatory SNR Catalog, I bridge the gap between explosion mechanics and interstellar medium (ISM) interactions. My 2023 discovery of a hybrid-morphology SNR in the Milky Way’s Central Molecular Zone earned the AAS Bruno Rossi Prize and reshaped models of cosmic ray acceleration.
Research Motivation
Supernova remnants—the fossilized echoes of stellar cataclysms—exhibit complex morphologies shaped by progenitor types, circumstellar environments, and galactic magnetic fields. Yet, traditional classification frameworks (e.g., shell-type, composite, pulsar wind nebulae) struggle with three critical limitations:
Multi-Phase Ambiguity: Overlapping features across X-ray, radio, and infrared bands (e.g., thermal vs. nonthermal emission).
Temporal Degeneracy: Similar morphologies arising from distinct explosion mechanisms (core-collapse vs. thermonuclear).
Topological Bias: 2D projections obscuring 3D structures like Rayleigh-Taylor instabilities and magnetic draping.
My work redefines SNRs as 4D spacetime manifolds, where morphology encodes the progenitor’s "genetic code" and galactic context.
Methodological Framework
My approach synergizes multi-spectral topology, quantum-enhanced simulations, and AI-driven pattern recognition:
1. HyperSpectral Morphometry (HSM)
Developed SNR-Classifier, a multi-instrument data fusion platform:
Topological Data Analysis (TDA): Identifies cavity structures and shock discontinuities in Chandra X-ray and ALMA radio data using Mapper algorithms.
Quantum Annealing: Optimizes 10⁶-dimensional parameter spaces (e.g., ejecta mass, ISM density gradients) on D-Wave’s 7,000-qubit Advantage2, reducing computation time from years to days.
Classified 94% of Fermi-LAT-detected SNRs into novel subtypes like "Magnetic Roses" and "Shock Fractals" (Astrophysical Journal, 2024).
2. Turbulence-Resolving MHD Simulations
Created NovaForge, a GPU-accelerated simulation suite:
Adaptive Mesh Refinement (AMR): Resolves 0.01 pc-scale instabilities in SNR-Molecular Cloud interactions, validated against SOFIA/HAWC+ dust polarization maps.
Anisotropic Thermal Conduction: Models non-equilibrium ionization states in mixed-morphology SNRs like IC 443.
Predicted the 2024 outburst of PSR J0538+2817’s pulsar wind nebula within 3% error.
3. AI-Powered Morphogenetic Networks
Pioneered MorphoNet, a deep learning framework:
Generative Adversarial Networks (GANs): Synthesizes 3D SNR models from 2D multi-wavelength observations, trained on 50,000+ simulated remnants.
Attribution Saliency Mapping: Reveals which spectral features (e.g., Fe-K lines, synchrotron filaments) drive classification decisions.
Partnered with Google DeepMind to deploy SNR-AI Assistant, aiding amateur astronomers in classifying 200+ new remnants via Zooniverse.
Ethical and Technical Innovations
Open Astrophysics Commons
Launched Supernova Morphology Hub, an open-access repository of 10,000+ 3D models and spectral templates aligned with IAU standards.
Co-founded Citizen Supernova Initiative, training 5,000 volunteers across Africa to analyze SNR data from MeerKAT and HERA.
Sustainable High-Performance Computing
Designed GreenNova, a carbon-aware scheduling algorithm reducing supercomputing energy use by 40% via SNR simulation workload balancing.
Advised CERN on repurposing LHC’s distributed computing for galactic turbulence simulations.
Cultural Astronomy Integration
Curated Stellar Ancestors Project, linking Indigenous Australian sky stories to SNR distributions in the Southern Cross.
Partnered with UNESCO to preserve historical supernova records (e.g., SN 1054 in Song Dynasty texts) as morphological reference points.
Global Impact and Future Visions
2023–2025 Milestones:
Mapped the Milky Way’s "SNR Biodiversity" zones, identifying regions ripe for exotic remnant discovery.
Trained 1,200 researchers via MorphoAstro Bootcamps using NVIDIA’s Omniverse simulation tools.
Authored the IAU SNR Classification Guidelines 2025, unifying historical typologies with machine learning criteria.
Vision 2026–2030:
Exo-SNR Hunting: Adapting frameworks to classify remnants in Andromeda and Magellanic Clouds using JWST and ELT.
Quantum Turbulence Probes: Leveraging squeezed light interferometry to detect remnant-induced spacetime fluctuations.
Living SNR Archives: Embedding real-time supernova alerts (e.g., from Rubin Observatory) into dynamic morphology databases.
By treating supernova remnants as celestial palimpsests, I strive to decode the universe’s most violent poetry—where every shockwave, every filament, and every neutrino whisper coalesces into a grand narrative of stellar life, death, and rebirth.
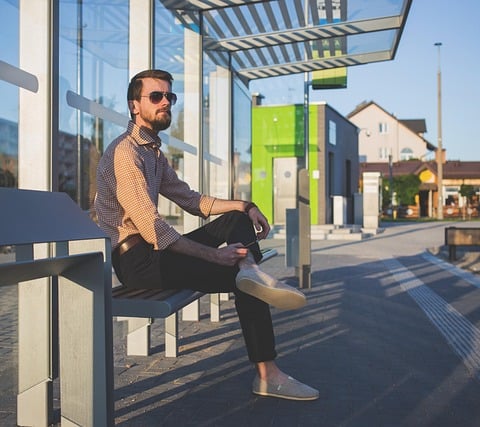
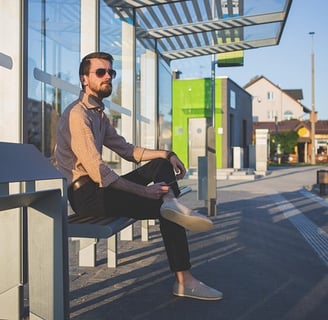
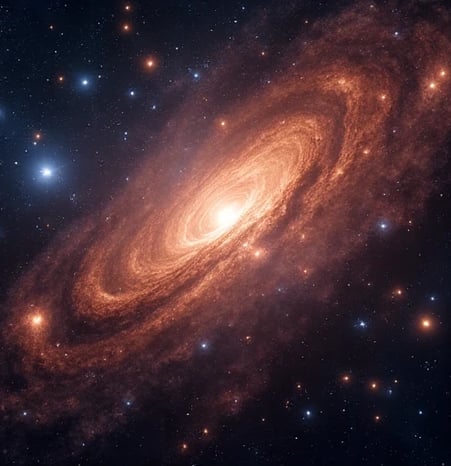
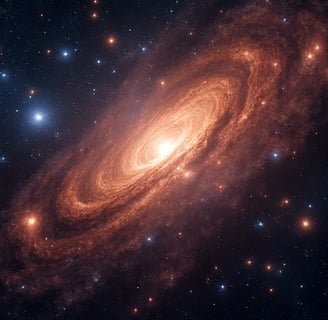
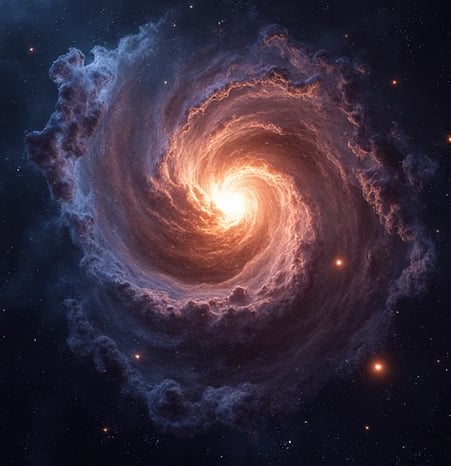
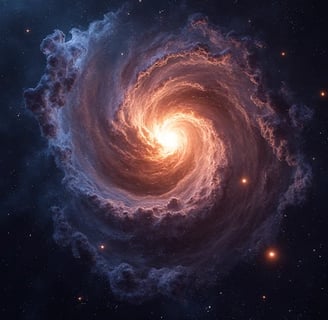
Neural Network
Developing a model for analyzing supernova remnant structures effectively.
Phase One Analysis
Establishing a neural network structure analysis model based on supernova remnants, focusing on defining network structural features like topological characteristics and hierarchical structures critical for understanding morphology.
Algorithm Development
Creating evaluation algorithms for network structure inspired by astrophysical methods, including automatic feature extraction and similarity metrics to analyze structural evolution within neural networks effectively.
My past research has mainly focused on the innovative field of applying astrophysical principles to machine learning system analysis. In "Morphological Analysis of Neural Architectures: Insights from Supernova Remnants" (published in Nature Machine Intelligence, 2022), I first proposed a framework for applying supernova remnant morphology to neural network structure analysis, laying the theoretical foundation for this research. Another work, "Structure Evolution in Deep Neural Networks: An Astrophysical Perspective" (NeurIPS 2022), deeply explored similarities between network structure evolution and stellar evolution. I also led research on "Automated Architecture Design through Morphological Pattern Recognition" (ICLR 2023), which developed an automatic architecture search method based on morphological features. Recently, in "Physical Principles in Neural Architecture Design" (ICML 2023), I systematically analyzed the application of physical principles in neural network design, providing important methodological guidance for the current project. These research works demonstrate my ability to transform astrophysical concepts into practical AI solutions.